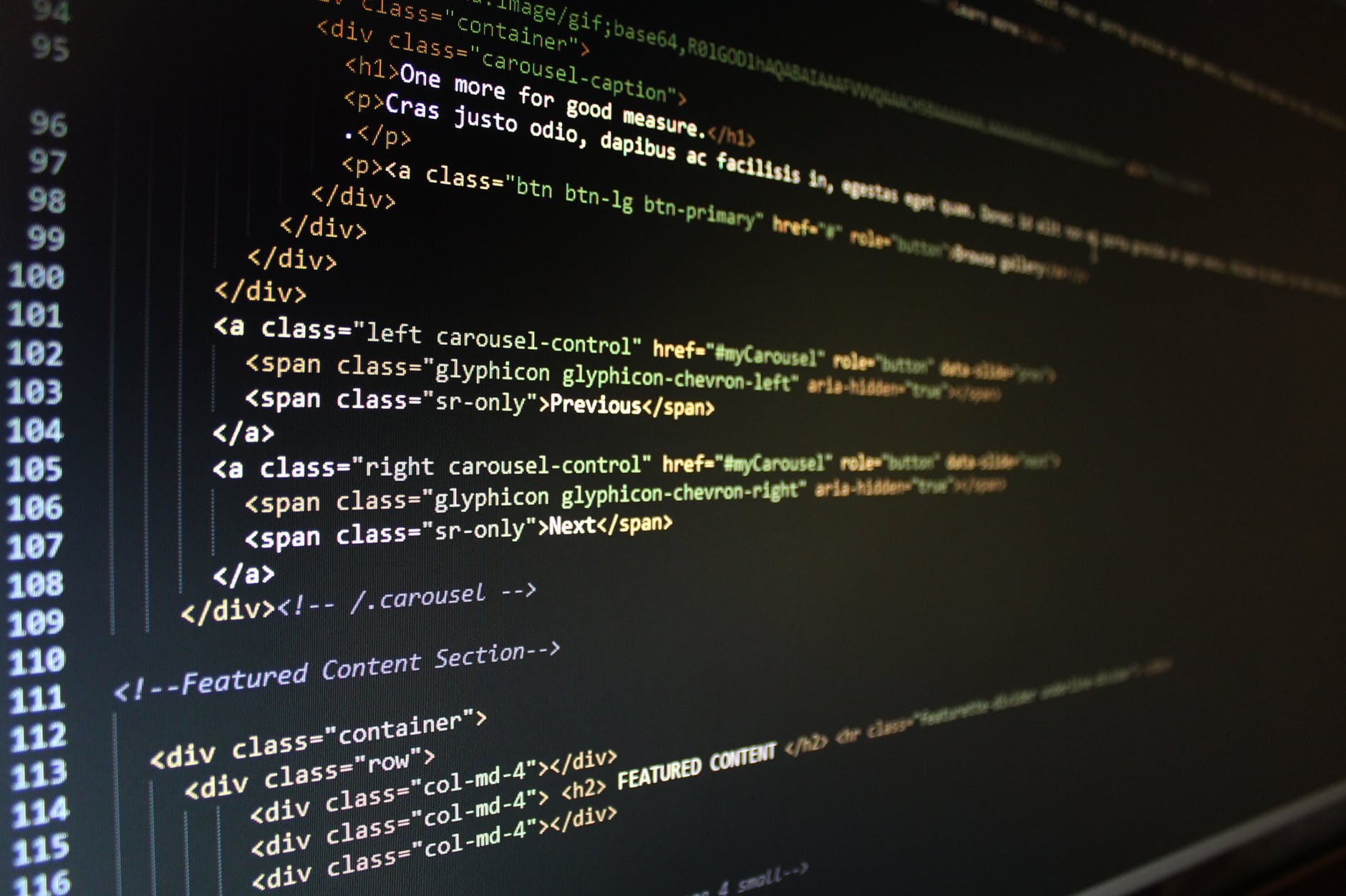
使用 .yml
檔案
- 將當前環境設置輸出為
environment.yml
,再用來創建配置一致的新環境
(base) ~$ conda activate current_env
(current_env) ~$ conda env export > environment.yml
(current_env) ~$ conda deactivate
(base) ~$ conda env create -n new_env -f environment.yml
- 更新現有環境
(current_env) ~$ conda env update -f environment.yml --prune
- 在
environment.yml
中對 pip 安裝指令加參數 [ref]
name: your_env
channels:
- conda-forge
- defaults
dependencies:
- ...
- pip:
- absl-py==0.15.0
- git+https://github.com/your_private_package
- your-package==1.2.3 -f <wheel_source_url>
prefix: /path/of/the/virtual/environment
使用 .txt
檔案
- 將當前環境設置輸出為
spec_file.txt
(base) ~$ conda activate current_env
(current_env) ~$ conda list --explicit > spec_file.txt
(current_env) ~$ conda deactivate
(base) ~$ conda create -n new_env -f spec_file.txt
更多 conda 指令: